The Key to Credible Impact Measurement?
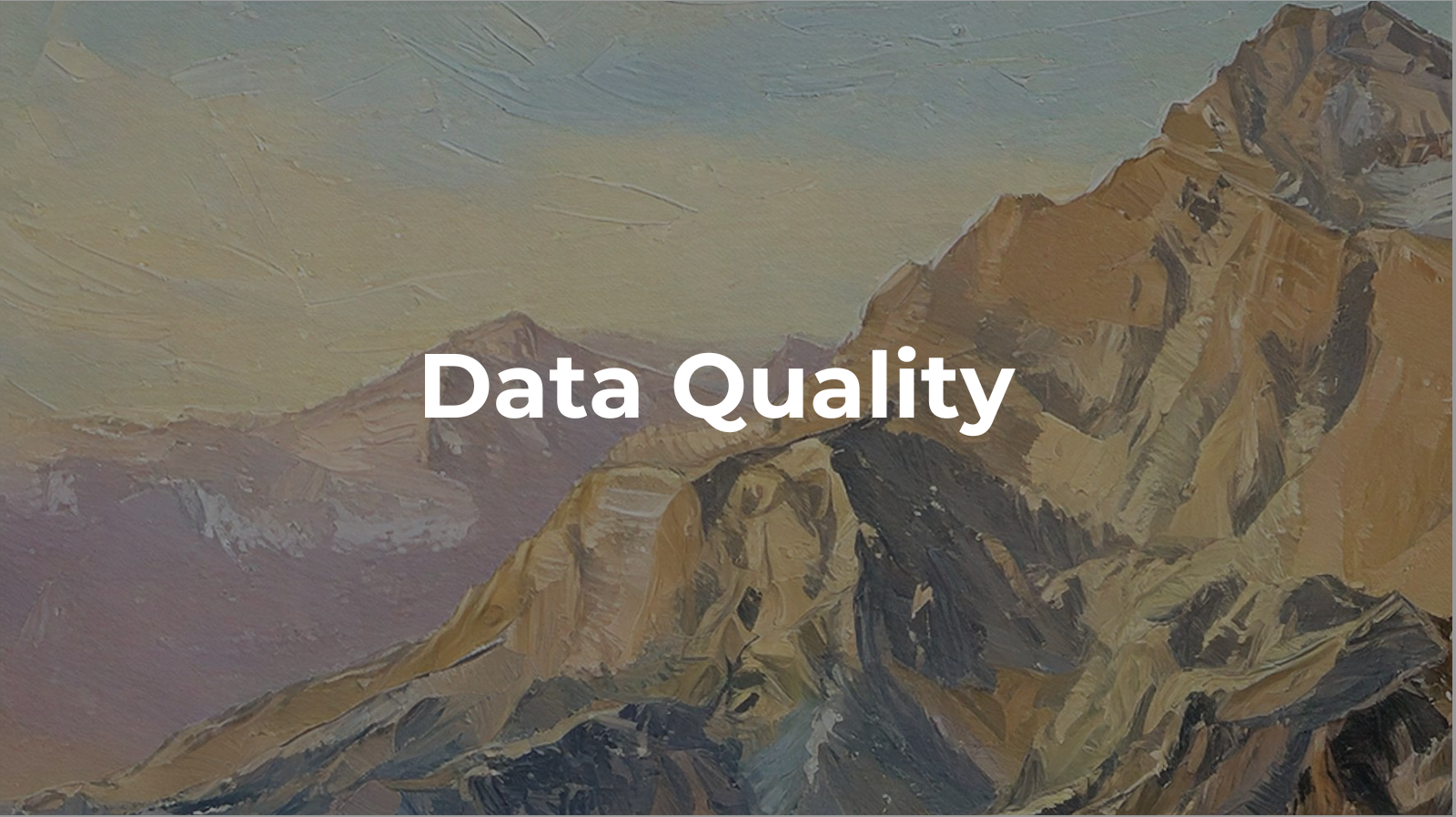
Imagine a world where every data point tells a story—of impact, progress, and change. But how do we know these stories are real? How can impact-driven organisations prove their efforts are making a difference? What if the numbers they rely on are flawed? Can we trust impact measurement when the data behind it is inconsistent or incomplete? And if data quality isn’t guaranteed, what does that mean for the future of meaningful, measurable change? Let’s dive into this, trying to answer the question
Is data quality just optional or existential for impact measurement and consequently for achieving sustainable change?
We interviewed seven impact investing funds about their data quality issues. Here is what they told us
The main feedback in conversations with impact investors is that data quality and availability is the biggest obstacle in impact measurement and reporting. After we heard the complaints over and over again we started a learning session as part of our R&D work to understand what that actually means. We talked to seven investment funds that manage a total of around 2 billion USD assets under management in impact investments. We asked openly “We often heard that data quality is bad. What exactly is the problem with data quality?”
Six out of the seven funds confirmed that data quality is a significant issue. They pointed out that inconsistent definitions and a lack of standardisation often lead to varied interpretations of key metrics, such as what qualifies as “employment” or how to measure income. Data availability is another concern, with many funds highlighting the scarcity of disaggregated data (e.g., gender-specific figures) and the inconsistent reporting of annual versus cumulative figures. Additionally, the process of data collection varies widely depending on who is gathering the information, whether it’s self-reported by companies or collected directly from beneficiaries, and limited validation procedures further undermine data reliability.
One fund summed it up well:
The lack of clear definitions and standardisation creates a situation where data comes from various sources with little consistency, ultimately compromising our ability to measure true impact.
With these challenges in mind, it becomes crucial to ask: What exactly makes data “high-quality”? Let’s explore the key dimensions that underpin robust and reliable impact measurement.
What Makes Data “High-Quality”? A summary on what science has to say about it.
In general, data quality is multidimensional. According to multiple studies (e.g. Batini et al. (2009)), it encompasses six dimensions: Completeness, Accuracy, Consistency, Validity, Uniqueness, Timeliness.
Building up on those, we started research on data quality criteria especially with regards to impact measurement. Based on our results we propose the following criteria as the most relevant for collecting high-quality data:
- Data Consistency: Ensuring Logical and Structural Reliability
Data consistency ensures that responses align with expected patterns, formats, and logical relationships. This involves verifying that answers are provided only when relevant (e.g., skipping questions that do not apply to a respondent), fall within predefined thresholds, align with other data points and adhere to specified formats. Without these checks, inconsistencies can render datasets unreliable.
Example: A nonprofit surveys farmers about their daily routines but fails to validate responses against expected ranges. One farmer reports working 18 hours per day, exceeding the predefined threshold of 2–16 hours. Without consistency checks, this outlier skews the dataset, leading to inaccurate conclusions about labor patterns or potential overwork.
Solution: To address this, organisations should implement automated validation rules to enforce skip logic and range limits, standardize data formats (e.g., structuring phone numbers uniformly), and conduct manual reviews to flag logical contradictions. - Data Representativity: Reflecting the Target Population
Representative data requires two key elements: ensuring the sample accurately mirrors the demographics, behaviors, or needs of the target population, and securing sufficient inclusion of predefined subgroups (e.g., gender, income brackets) to avoid skewed insights. Without this, datasets risk overlooking marginalized voices and misdirecting resources.
Example: Consider a GreenTech startup surveying farmers exclusively via a mobile app. This approach excludes 40% of their target population—elderly or low-income farmers without smartphones—while also underrepresenting women, who constitute only 40% of respondents despite comprising half the farming community. The resulting data skews toward tech-savvy male farmers, leading to overinvestment in digital tools rather than low-tech irrigation solutions needed by excluded groups.
Solution: To ensure representativity, organisations should adopt stratified sampling to meet quotas for critical subgroups and combine digital tools with in-person interviews to reach offline populations. Regular demographic audits during data collection can further prevent gaps.
- Data Integrity: Verifying Respondent Authenticity
Data integrity focuses on validating the trustworthiness of individual responses by confirming realistic participant profiles (e.g., valid phone numbers and plausible names), assessing interview quality (e.g., duration, enumerator observations), and ensuring methodological transparency (e.g., disclosing paid participation). Compromised integrity risks misinformation and erodes stakeholder trust.
Example: For instance, a social enterprise using paid online surveys might encounter falsified responses: e.g. 30% of entries share identical phone numbers, and 20% of interviews are completed in under 2 minutes (far below the expected 10-minute average). Enumerators might also note participants rushing through questions or providing implausible answers, such as listing a single repeated digit as a phone number.
Solution: To safeguard integrity, organisations should cross-verify contact details, monitor interview duration, embed attention-check questions, and train enumerators to flag suspicious behavior. Auditing incentive structures (e.g., paid vs. unpaid participation) and maintaining clear records of data collection methods also enhance accountability.
Why Does Data Quality Matter for Impact Measurement?
Impact measurement is only as credible as the data fueling it. Poor-quality data isn’t just a technical hiccup—it’s a threat to mission success, donor trust, and societal progress. We recognise that data quality is the foundation of holism, comparability, trust, and accountability in impact measurement. Without accurate data, efforts to assess social and ecological outcomes become unreliable, leading to misguided decisions and wasted resources.
How Data Quality Drives Social Impact
Reliable data ensures that organisations can measure progress effectively, adapt or pivot strategies, and maximise impact. Poor data, on the other hand, distorts reality, perpetuates inequities, and undermines stakeholder trust. Robust data governance, continuous monitoring, and advanced analytics enable organisations to uncover actionable insights, ensuring that social initiatives are truly impactful.
In practice, transforming your organisation’s data quality begins with clear, actionable steps. For social enterprises, consider these four concrete measures:
- Establish a Solid Foundation:
Utilise standardised and widely acknowledged indicators to ensure comparability across datasets. Concentrate on identifying and tracking the most important key performance indicators (KPIs) that align with your mission.
- Implement Robust Data Collection Processes:
Integrate comprehensive data collection methods into your day-to-day operations and customer success management. This approach ensures that every interaction is captured accurately, minimising manual errors and enhancing data consistency.
- Utilise the Right Tools for Data Processing:
Invest in a robust digital data pipeline and adopt best practices in data management. Employ automated tools and establish rigorous quality checks to detect and correct inconsistencies in real time, ensuring your data remains reliable.
- Incorporate Data into Management Meetings:
Regularly bring data insights into your strategic discussions. By reviewing results frequently, you can make actionable decisions, promptly address any discrepancies, and continually refine your approach for improved outcomes.
New technologies like machine learning can predict, audit, and resolve data quality risks, but human oversight is essential to prevent inequities. Let’s have a look at how, through continuous R&D, we have developed a great solution for our customers.
leonardo intelligence powers automated data quality audits: Using AI to Ensure Data Quality
The leonardo intelligence runs AI-powered audits, ensuring data quality and reporting credibility. ensuring data quality and reporting credibility.
Our data science team under the lead of Alan Sicart has developed the first version of leonardos data quality audit, designed to maximize data quality while minimizing collection burdens.
We want to maximize data quality while minimizing data collection efforts. That means understanding whether a particular question—or even an entire respondent—deserves review, and determining the minimum amount of clean data required to represent our target population.
Alan Sicart, Lead Data Scientist, leonardo. impact
How It Works
Traditional rule-based checks often miss subtle data flaws, especially in small or complex datasets. Our automated audit addresses this by:
- Learning from Patterns: Standard machine learning models analyze large datasets to detect outliers (e.g., implausible working hours) and inconsistencies (e.g., mismatched family size vs. children).
- Leveraging LLMs for Small Samples: When datasets are too small for reliable patterns, we use Large Language Models (LLMs) to interpret unstructured responses (e.g., validating open-ended feedback or identifying rushed survey answers).
- Prioritizing Risks: The system flags high-risk data (e.g., non-representative samples or suspicious respondents) for human review, reducing wasted effort on clean datasets.
If you want to learn more about our AI-powered data quality audits, we would be happy if you contact us.
Conclusion
Impact measurement isn’t just about collecting data—it’s about ensuring that data reflects reality. By prioritising data quality, social organisations can unlock funding, strengthen donor trust, and influence policy, transforming raw data into powerful insights that drive sustainable, scalable change. High-quality data empowers organisations to align actions with values, inspire collective action, and drive systemic change. Thus it is existential for impact measurement and consequently for achieving sustainable change.
Want to know more?
Get in touch with us and and start to measure impact confidently.